RidgeClassifier#
Implementa un clasificador usando regresión Ridge
En la regresión ridge, los coeficientes del modelo minimizarn la suma penalizada de residuales al cuadrado:
\min_w ||Xw -y||_2^2 + \alpha ||w||_2^2
con \alpha \ge 0
Este modelo impone una penalización al tamaño de los coeficientes.
La penalización se aplica únicamente sobre los coeficientes de x.
Para adaptar el modelo de regresión al problema de clasificación, primero se convierte y en \{-1, 1\}, y luego resuelve el problema usando regresión.
[1]:
import matplotlib.pyplot as plt
from sklearn.datasets import make_blobs
NPOINTS = 150
X, y_true = make_blobs(
n_samples=NPOINTS,
n_features=2,
centers=3,
cluster_std=0.8,
shuffle=False,
random_state=12345,
)
plt.scatter(X[:50, 0], X[:50, 1], color="red")
plt.scatter(X[50:100, 0], X[50:100, 1], color="blue")
plt.scatter(X[100:, 0], X[100:, 1], color="green")
plt.gca().set_aspect("equal", adjustable="box")
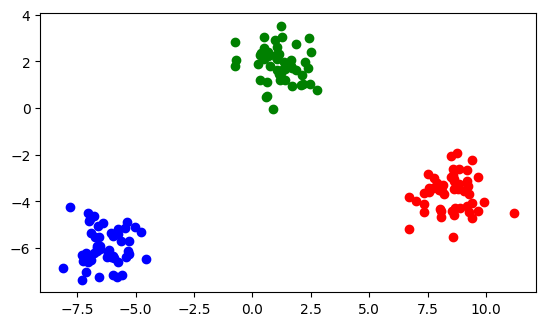
[2]:
from sklearn.linear_model import RidgeClassifier
ridgeClassifier = RidgeClassifier(
# ---------------------------------------------------------------------
# Regularization strength; must be a positive float. Regularization
# improves the conditioning of the problem and reduces the variance of
# the estimates. Larger values specify stronger regularization. Alpha
# corresponds to 1 / (2C) in other linear models such as
# LogisticRegression or LinearSVC.
alpha=1.0,
# ---------------------------------------------------------------------
# Whether to fit the intercept for this model.
fit_intercept=True,
# ---------------------------------------------------------------------
# Maximum number of iterations for conjugate gradient solver.
max_iter=None,
# ---------------------------------------------------------------------
# Precision of the solution. Note that tol has no effect for solvers
# ‘svd’ and ‘cholesky’.
tol=1e-4,
# ---------------------------------------------------------------------
# Solver to use in the computational routines:
# * ‘auto’ chooses the solver automatically based on the type of data.
# * 'svd'
# * 'cholesky'
# * 'sparse_cg'
# * 'lsqr': regularized least-square
# * 'sag': Stochastic Average Gradient
# * 'lbfgs'
solver="auto",
# ---------------------------------------------------------------------
# When set to True, forces the coefficients to be positive.
positive=False,
# ---------------------------------------------------------------------
# Used when solver == ‘sag’ or ‘saga’ to shuffle the data.
random_state=None,
)
ridgeClassifier.fit(X, y_true)
ridgeClassifier.predict(X)
[2]:
array([0, 0, 0, 0, 0, 0, 0, 0, 0, 0, 0, 0, 0, 0, 0, 0, 0, 0, 0, 0, 0, 0,
0, 0, 0, 0, 0, 0, 0, 0, 0, 0, 0, 0, 0, 0, 0, 0, 0, 0, 0, 0, 0, 0,
0, 0, 0, 0, 0, 0, 1, 1, 1, 1, 1, 1, 1, 1, 1, 1, 1, 1, 1, 1, 1, 1,
1, 1, 1, 1, 1, 1, 1, 1, 1, 1, 1, 1, 1, 1, 1, 1, 1, 1, 1, 1, 1, 1,
1, 1, 1, 1, 1, 1, 1, 1, 1, 1, 1, 1, 2, 2, 2, 2, 2, 2, 2, 2, 2, 2,
2, 2, 2, 2, 2, 2, 2, 2, 2, 2, 2, 2, 2, 2, 2, 2, 2, 2, 2, 2, 2, 2,
2, 2, 2, 2, 2, 2, 2, 2, 2, 2, 2, 2, 2, 2, 2, 2, 2, 2])
[3]:
ridgeClassifier.score(X, y_true)
[3]:
1.0
[4]:
ridgeClassifier.coef_
[4]:
array([[ 0.15400142, -0.14156887],
[-0.11087184, -0.14212033],
[-0.04312957, 0.2836892 ]])
[5]:
ridgeClassifier.intercept_
[5]:
array([-0.87017324, -0.57474307, 0.44491631])